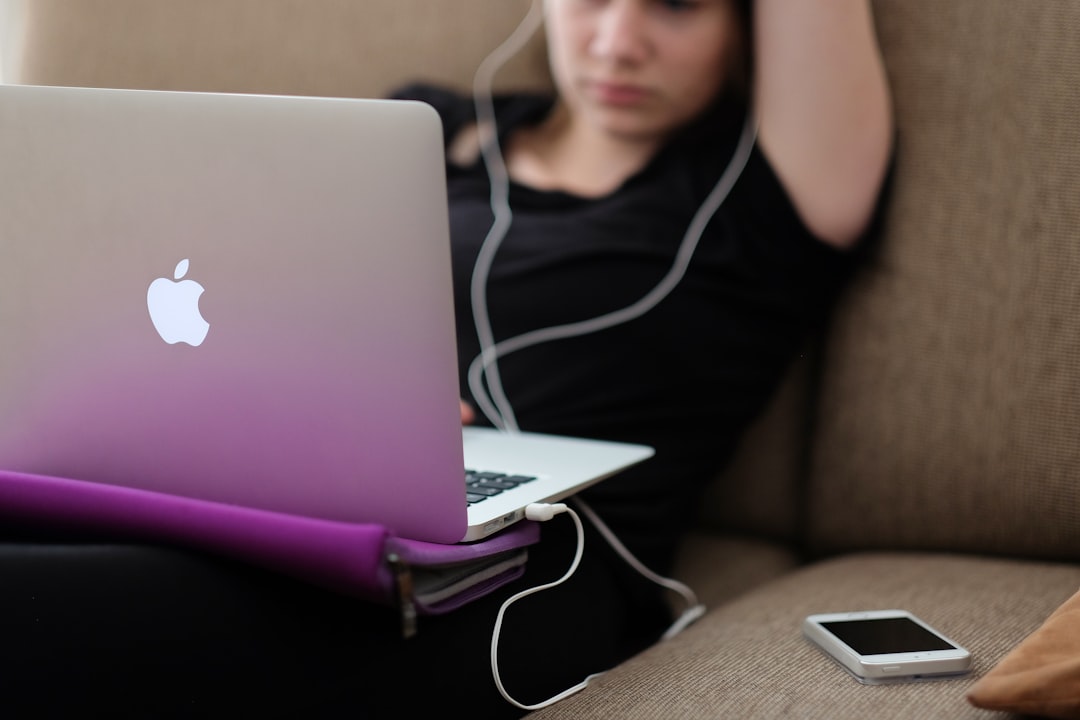
Unlocking the Power of Reinforcement Learning with Open-Source Frameworks
Accelerating Reinforcement Learning with Open-Source Frameworks Introduction Reinforcement learning (RL) has emerged as a crucial area of research in machine learning, with applications in robotics, game playing, and autonomous driving. However, RL experimentation can be computationally expensive and time-consuming. This article will explore how open-source frameworks can accelerate RL experimentation, making it more efficient and accessible to researchers and practitioners. Prerequisites Basic understanding of reinforcement learning concepts (e.g., agents, environments, policies) Familiarity with Python programming language Experience with deep learning frameworks (e.g., TensorFlow, PyTorch) Setting Up the Environment Popular Open-Source RL Frameworks Several popular open-source RL frameworks can help accelerate RL experimentation: ...